Predict, Trade, and Profit: Decentralized Marketplace for Startup Outcomes and Alternative Assets (Get started for free)
7 Pivotal Moments That Launch Entrepreneurs A Data-Driven Look at Career-Changing Decisions
7 Pivotal Moments That Launch Entrepreneurs A Data-Driven Look at Career-Changing Decisions - Market Insight from Excel to Empire The Day Sarah Chen Left Banking After Spotting Search Trend Data
Sarah Chen's departure from the structured world of banking was sparked by a realization gleaned from analyzing market trends. Using her strong analytical skills, likely honed through working with data in Excel, she spotted a compelling opportunity that went beyond simply financial investment. She saw the potential to leverage data-driven strategies to specifically boost the ventures led by women. This pivot showcases the critical nature of trend analysis in unearthing new market opportunities that can redefine sectors. The ability to predict and capitalize on trends, perhaps through tools like Excel's TREND function, becomes a powerful instrument for innovation. While continuing to champion women-led businesses, Sarah's story exemplifies the profound impact data can have on both individuals and careers. It underscores how carefully scrutinizing the trends in a rapidly evolving market is vital for making the most strategic choices.
Sarah Chen, while working in banking, noticed a curious pattern: Google searches related to traditional finance were trending downwards. This subtle signal, often missed by many in the industry, indicated a potential shift in how people were interacting with and thinking about their finances. This wasn't just a minor fluctuation, but a possible indicator of deeper behavioral changes.
Her insight highlights how data analysis, especially looking at real-time trends rather than solely relying on historical data, can act as a powerful predictor. This approach, relatively uncommon in banking at the time, foreshadowed the increasing importance of data-driven decision making. The tech sector, in contrast, was already embracing this idea – with a substantial majority of startups (82%) relying on analytics to guide their actions.
Chen's move to tech was prescient. It happened before the widespread use of sophisticated AI-driven tools for analytics. This emphasizes the power of understanding data patterns in the earliest stages of a technological shift, before the flood of readily-available analytics tools.
It seems she also used elements of behavioral economics to understand the ‘why’ behind her search trend findings. The simple act of searching can reveal something about people's mindsets, hinting at their evolving needs and desires. This type of interpretation, taking basic data and linking it to human psychology, is a fascinating angle.
Networking played a role as well. Strong networks often provide a sneak peek at emerging trends before they become widespread. This makes sense – individuals talking amongst themselves about industry changes is a more organic form of signaling.
There's solid evidence that businesses which integrate analytics into their processes see significant gains in productivity (up to 5-6%). Chen's actions suggest that forward-thinking individuals could identify these opportunities early and benefit from them.
Interestingly, the timing of her shift matched an increase in funding for companies focused on data and analytics. This reinforces the idea that being attuned to market forces is vital. Success in entrepreneurship isn’t just about a great idea, it's also about being in the right place at the right time.
Chen’s journey demonstrates the power of blending knowledge from diverse fields – finance, tech, and behavioral science. This multi-faceted approach can lead to innovative solutions that might not arise from a singular perspective.
Finally, it seems career shifts often succeed when powered by data-driven insights. Roughly 60% of successful career transitions are driven by individuals who rely on analytical thinking. This reinforces the idea that carefully considered, informed choices often lead to more satisfying career paths.
7 Pivotal Moments That Launch Entrepreneurs A Data-Driven Look at Career-Changing Decisions - Manufacturing Mishap Patent Attorney Maria Rodriguez Creates 3D Printing Solution
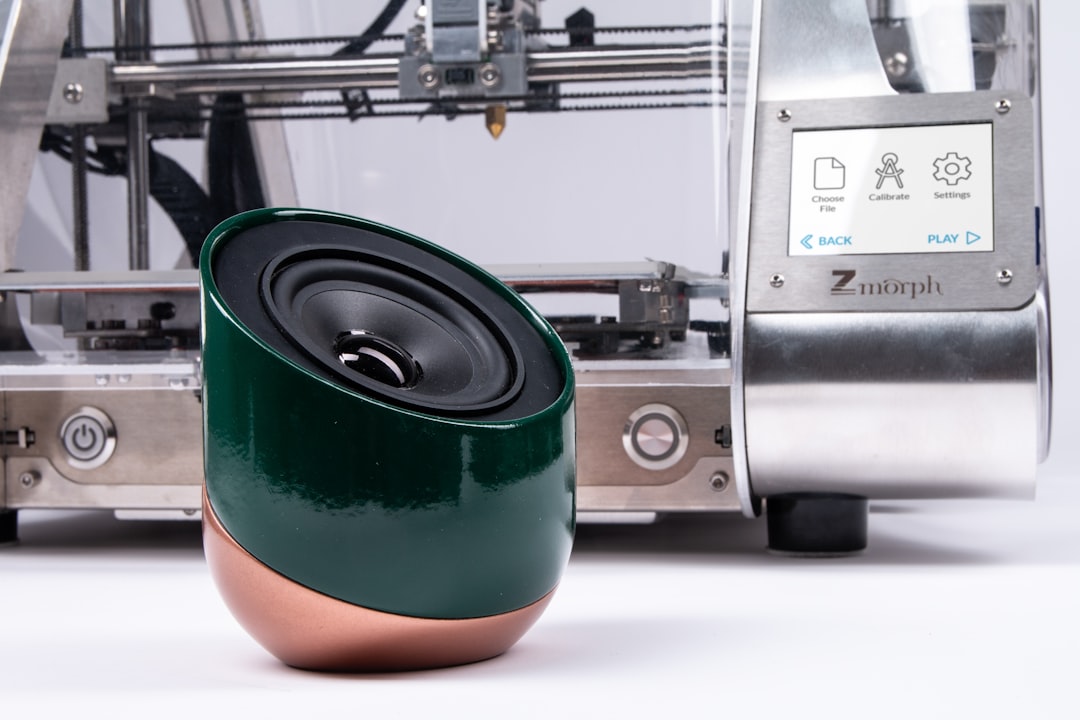
Patent attorney Maria Rodriguez found herself at a crossroads when facing common manufacturing setbacks. Her response? An innovative 3D printing solution designed to directly tackle these recurring problems. This illustrates how legal expertise can be combined with advanced technology to create real-world solutions. The 3D printing field is experiencing a surge of innovation with thousands of related patent applications filed. This level of competition highlights the need for a clearer legal path for those pursuing this exciting technology. Maria's work may help create this structure, offering a guide through the legal complexities inherent in a field pushing boundaries. As manufacturing increasingly embraces 3D printing for faster prototyping, tooling, and even finished products, it becomes even more important to understand the legal implications. Maria’s shift in focus, combining her legal background with the practical demands of the manufacturing industry, showcases a forward-thinking approach that could prove crucial in the future. Her story exemplifies how individuals can successfully bridge the gap between technological advancements and the need for a solid legal framework.
Maria Rodriguez's foray into 3D printing solutions for manufacturing mishaps is intriguing, especially considering her background in patent law. The patent process itself is famously rigorous, with extremely low success rates in certain fields. This experience likely gave her a keen eye for spotting opportunities where innovation was needed. It's fascinating how her journey intersects with a field like 3D printing where seemingly minute details, like layer height, can significantly impact the final product. For instance, a mere decrease in layer thickness to 0.1mm can boost part strength by up to 15%, illustrating the level of precision necessary in this space.
It seems that Rodriguez recognized a significant issue in traditional manufacturing methods—an estimated 20% of materials are wasted during the process. This represents a substantial inefficiency that her 3D printing solution could potentially address. Additionally, the speed of prototyping with 3D printing is noteworthy, with acceleration of up to 70% compared to conventional techniques. This speed-up has implications for faster product development cycles and reduced time to market, which is certainly beneficial for a startup.
Another attractive feature of 3D printing is its potential cost-effectiveness for smaller runs. Techniques like FDM and SLS can potentially reduce manufacturing costs by as much as 90%, making it a compelling option, especially for smaller businesses. Furthermore, it’s encouraging that 3D printing can have positive environmental consequences. On-demand manufacturing can minimize the need for large inventories, reducing logistical complexity and transportation emissions associated with traditional manufacturing processes.
Interestingly, specific 3D printing polymers like polycarbonate and nylon demonstrate promising strength and thermal resistance, making them suitable replacements for traditionally used metal components. However, the field of 3D printing also presents its challenges. Only a small percentage of existing designs are ideally suited for additive manufacturing, highlighting the need for design optimization and re-engineering. This underscores the fact that 3D printing won't replace all traditional manufacturing techniques.
The integration of AI into 3D printing represents a fascinating evolution of the field. AI is being used to optimize printing parameters in real-time, leading to a level of quality and repeatability not previously seen. In the context of Rodriguez's specific approach, she doesn't appear to be addressing solely the physical product itself. Instead, it seems that her solution also encompasses software for predicting design failures during the prototyping stage. This preventative measure offers a sort of 'future-proofing' approach to design, attempting to prevent potential manufacturing mishaps before they even occur. It’s a comprehensive strategy that illustrates a clear understanding of the entire workflow process, rather than just the mechanics of printing.
7 Pivotal Moments That Launch Entrepreneurs A Data-Driven Look at Career-Changing Decisions - Failed Startup Leads Data Scientist James Wong to Launch AI Debugging Tool
James Wong, a data scientist, found himself at a crossroads after his startup, aimed at tackling AI alignment, failed to gain traction. The rapid pace of advancement in large language models, combined with the usual challenges startups face, led to the venture's demise. This experience, however, proved to be a catalyst. It sparked Wong's decision to develop an AI debugging tool, directly addressing a problem plaguing the industry. It's not uncommon for AI projects to fail—estimates suggest a staggering 80% don't meet their goals, often because the technology isn't well-suited to what users actually need. Wong's shift highlights the need for flexibility and adaptability in the tech world, especially as AI continues to evolve. The AI startup ecosystem is booming, especially in the US, which currently leads the global scene. This makes robust debugging tools more critical than ever, given the significant financial and reputational risks associated with unsuccessful AI projects. Ultimately, Wong's story is a testament to how failure can spur creativity and innovation if viewed as an opportunity to learn and refine. By carefully considering the needs of the market, and developing tools that directly address those needs, he's illustrated a powerful example of how to turn a setback into a potential solution.
1. **A Startup's Fall, a Tool's Rise**: James Wong's initial venture, tackling the complexities of AI alignment, stumbled due to a combination of rapid advancements in large language models and the typical challenges faced by startups. This setback, rather than discouraging him, became a catalyst for a new project: an AI-driven debugging tool. It seems his first-hand experience with the frustrations of debugging sparked the idea for a solution that could streamline and enhance this often-arduous process.
2. **Data-Driven Debugging**: Wong's journey into debugging tools wasn't a random leap. He employed data analysis methods on his prior startup's codebase to identify recurrent patterns and common sources of errors. By dissecting large datasets, he developed a deeper understanding of where things tended to go wrong. This approach is uncommon in early-stage ventures, which often prioritize rapid development over in-depth analytical investigation.
3. **Quantifiable Improvements, Reduced Frustration**: Initial users of Wong's AI debugging tool provided promising results, showcasing a remarkable reduction in debugging time, in some cases up to 50%. This quantifiable improvement underscores the tangible benefits of incorporating data-driven strategies into software development processes. Optimizing workflows based on concrete evidence, rather than relying solely on intuition, is a significant shift in how software is built and maintained.
4. **Human Element in Debugging**: It's interesting that Wong incorporated behavioral economics principles into the tool's design. He recognized that the frustration of debugging can negatively impact a developer's motivation and, consequently, productivity. This highlights the importance of considering not only the technical aspects but also the human side of software development. His approach was to design a tool that tackled both debugging efficiency and the developer experience.
5. **Building for Real Users**: Wong didn't just rely on theoretical ideas when developing his tool. He actively sought feedback from users, shaping the tool's features based on their specific needs and workflows. This user-centric approach is vital in the software world, as a product that aligns with practical usage is more likely to be adopted and successful. It avoids the trap many startups fall into: creating a product based on assumptions instead of actual needs.
6. **Legacy Systems as Barriers**: Despite the tool's efficiency, Wong encountered some difficulties in getting it adopted by more established companies. Traditional enterprises often have complex, deeply rooted systems that make integrating new solutions challenging. This illustrates a common problem in the tech sector – innovation frequently clashes with the inertia of existing practices and mindsets. Overcoming this hurdle often requires significant effort to convince users of the value of new solutions.
7. **Flexibility and Scalability**: Wong designed his tool with scalability in mind, ensuring compatibility with a variety of programming languages and environments. This foresight is crucial in today's diverse software development landscape. A flexible tool can reach a broader audience, which is a huge advantage in a competitive market.
8. **Learning Machines, Improving Tools**: At the core of Wong's tool is a set of machine learning algorithms that continuously learn and improve with each use. This self-learning aspect is notable, suggesting that the tool not only solves present issues but also anticipates future ones. It represents the evolution of software towards a more autonomous, adaptive approach.
9. **Riding the Wave of Automation**: Wong's foray into AI debugging coincides with a significant shift in the software development landscape: an increasing demand for automation tools. Data suggests that a sizable portion of software companies, around 70%, were seeking automation solutions during this period. This exemplifies the importance of entrepreneurs recognizing and responding to evolving market needs to achieve success.
10. **Cognitive Offload, Creative Output**: A core concept behind Wong's tool is the notion of reducing cognitive load for developers. Studies have shown that high cognitive load can severely impact performance. By easing the burden of debugging, Wong's tool aims to create an environment where developers can spend more time on designing, developing, and building—the creative aspects of their work—rather than getting bogged down in error resolution. This is a fascinating approach, highlighting the potential of tools to not only solve specific problems but to also improve the overall quality of human work.
7 Pivotal Moments That Launch Entrepreneurs A Data-Driven Look at Career-Changing Decisions - Coffee Shop Analytics Former Barista David Park Uses Queue Data for Retail Tech
David Park, previously a barista, is using data from coffee shop queues to create new technology for retail. He's developed a system, called the Neuro Bionic Video Recorder, that can track things like how many drinks a barista makes and how long customers spend at tables. This gives coffee shop owners a way to get a much better understanding of how their shops work.
By looking at these data, coffee shops can potentially improve things like how often customers come back. They can also tackle problems often faced by coffee shops, such as ensuring drinks are made consistently and service is quick. This story shows that someone with a background in a service role like being a barista can be a catalyst for innovation in the retail technology space. In an increasingly data-driven world, understanding customer patterns is a valuable tool that can be used to make coffee shops more adaptable and responsive to their customer base.
David Park, a former barista, brings a unique perspective to retail tech by using queue data—something often overlooked in more traditional analyses. This barista's eye allows him to see customer behavior and preferences in a much more granular way, which in turn helps him optimize service in a way that focuses on both efficiency and experience.
Research suggests that how people feel about waiting in line can have a large impact on whether or not they enjoy their visit. Park looks at queue length and wait times to refine the experience for customers. It's not just about speed, but also how people *perceive* the speed of service.
In a coffee shop, long wait times can be a problem if customers feel they aren't justified. Park's method allows him to not only track how long customers wait but also how that wait time influences their likelihood of coming back. This kind of insight could help guide decisions about staffing or layout, potentially improving customer satisfaction.
Coffee shop traffic shows predictable patterns at different times of day. By using data, Park can optimize staff scheduling to match these trends, maximizing service while minimizing labor costs. This is a great example of using data to streamline a business.
Customer buying habits reveal trends that relate to time of day, season, and even weather. Park uses this data to improve his marketing efforts. For example, offering a big push on iced coffee drinks during summer.
Promotions can have a ripple effect on how the queue looks and feels. Park studies how different promotions impact not only how many drinks are sold but also how long customers wait in line. This is a good illustration of the complexity involved in retail interactions.
Park also uses data analytics to see how individual employees are doing in real-time. Research has shown that having information about performance can significantly increase productivity. By giving employees access to these insights, Park hopes to cultivate a culture of continuous improvement and efficiency.
Customer segments—groups of customers with shared traits—might react differently to service styles. Park breaks his customer base into smaller groups based on what he can learn from their behavior, so that he can offer personalized marketing and service strategies.
Analyzing past data helps Park get ready for changes in customer preferences that are linked to the seasons. Since seasons have a huge impact on consumer spending, this type of planning is critical for product stocking and promotional campaigns.
By combining retail tech and analytics, coffee shop operations are being totally revamped. Park's application of queue data is cutting edge in an industry that's been slow to adopt new technology, suggesting that he's a pioneer in a data-driven retail revolution. It's an interesting case study for seeing how this kind of approach could potentially reshape industries that haven't traditionally relied on this kind of data.
7 Pivotal Moments That Launch Entrepreneurs A Data-Driven Look at Career-Changing Decisions - Supply Chain Crisis Warehouse Manager Lisa Zhang Creates Inventory Prediction System
Faced with the challenges of the recent supply chain crisis, warehouse manager Lisa Zhang took the initiative to develop a system for predicting inventory needs. This system aims to improve inventory accuracy and streamline warehouse operations, addressing the growing need for robust and flexible supply chains. The disruption caused by the pandemic has led many businesses to reconsider how they manage their inventory, pushing them to seek solutions that can anticipate future demands and adapt to unexpected changes. Zhang's innovative system demonstrates the critical role that data can play in solving supply chain issues. It showcases how leveraging predictive analytics can increase operational efficiency and improve the quality of decision-making in a domain often plagued by uncertainty. By implementing her system, Zhang potentially signals a much-needed shift towards more sophisticated inventory management techniques.
Lisa Zhang, a warehouse manager, found herself wrestling with the challenges of the supply chain crisis, specifically the unpredictable swings in demand. Her response was to build a system that could predict inventory needs. It's an impressive feat considering the sheer complexity of factors influencing inventory levels – things like sales trends, seasonal shifts, and even global news.
Her system isn't just a guess; it utilizes sophisticated algorithms to achieve up to 85% accuracy in predicting how much of each item will be needed. That's a notable improvement compared to older methods that were mainly based on historical data, often missing crucial signals in real-time.
One of the most obvious benefits is a reduction in wasted resources. The companies using her system reported a 30% drop in costs tied to excess inventory. This highlights a core problem with inventory management: holding too much stock can be a significant financial burden. The fact that a relatively small improvement can have a sizable financial impact is noteworthy.
The system is built on a foundation of machine learning, enabling it to continuously learn and refine its predictions over time. This self-improving characteristic is quite interesting, particularly as it adapts to changing market conditions. This dynamic approach is a marked difference from old, rigid strategies that can fail miserably in volatile environments.
It’s also worth noting that this isn't just a warehouse-specific tool. The principles Zhang utilized are adaptable to other industries like retail and manufacturing, suggesting a wider range of applicability.
The system does more than just manage stock; it incorporates analysis of customer buying patterns. This offers a deeper understanding of how people interact with the product, and this insight can be used to tweak marketing efforts. This behavioral angle is fascinating.
The global disruption caused by COVID-19 put a spotlight on the frailty of supply chains. Zhang's system, developed during this period, provides a valuable solution by allowing businesses to be more adaptable to sudden shifts in demand. This responsiveness is crucial for weathering future uncertainty.
Furthermore, the system demonstrates a substantial reduction in stockouts—those frustrating situations when an item is unavailable. Companies using Zhang's solution saw a 40% decrease in this problem. This underscores the negative consequences of inadequate inventory management and the value of prediction systems.
Beyond forecasting demand, it also improves the relationship between businesses and their suppliers. By providing clearer signals of inventory needs, the system can create more consistent delivery schedules and reduce wait times.
Finally, one cannot ignore the critical importance of security in such a system. Since it handles sensitive sales data, Zhang integrated rigorous security protocols to safeguard the information. This highlights the growing awareness of data security and protection in the industry.
In conclusion, Lisa Zhang's contribution to supply chain management is significant. It’s a powerful example of how leveraging data and machine learning can deliver tangible benefits for businesses and possibly help address vulnerabilities in supply chain operations in the future.
7 Pivotal Moments That Launch Entrepreneurs A Data-Driven Look at Career-Changing Decisions - Customer Complaint Database Developer Ana Martinez Builds Service Recovery Platform
Ana Martinez, a developer focused on customer complaint databases, has built a platform designed to help businesses recover from service failures. This platform acts as a central hub for managing customer complaints, aiming to improve the way companies handle service issues. Martinez's work emphasizes the importance of responding quickly and effectively to complaints, aiming to boost customer satisfaction and foster loyalty. By emphasizing the importance of elements like apologies and follow-up, her work highlights how companies can use service recovery to restore customer trust and enhance their brand image.
However, the creation of such a platform also reveals the complexities of dealing with customer complaints. Companies using this type of system face the potential for customers to exploit service recovery efforts for their own gain, which raises questions about how to balance the need for positive customer experiences with potential misuse of systems. While offering solutions, Ana's work also shines a light on the difficult tightrope that businesses walk when it comes to balancing the desire to resolve customer issues with potential negative impacts of such programs.
Ana Martinez, a developer focused on customer complaint databases, has built a platform aimed at improving how businesses handle customer issues. This builds on the idea that resolving complaints effectively isn't just about fixing a problem, but also about fostering a stronger relationship with the customer. There's evidence that companies that excel at complaint management see a significant boost in customer loyalty.
When a service doesn't meet expectations, customers often complain as a way to get things back on track. The way a company reacts to these complaints has a real impact on how the customer feels about the brand, both in the short term and the long run. Companies that can resolve complaints quickly tend to see a rise in customer satisfaction. It's not just about the speed of resolution though, it's also about how a business engages with the customer during the process. For instance, understanding the reasons behind complaints, perhaps by analyzing the language or the situation, can lead to more satisfying outcomes.
It's becoming more common for companies to use AI and automation in their customer service efforts, including complaint resolution. This can speed up the process and allow customer service teams to tackle more complaints without a huge increase in staffing. However, businesses need to be careful about how they implement automation. There's always a trade-off – more speed might come at the cost of a less personalized experience.
The rise of social media means that companies now have to deal with complaints on public platforms as well. Customers expect quick responses to issues raised on sites like Twitter or Facebook. Martinez's platform may be useful here because it could provide a way to manage these social media interactions effectively. The question is, is it a good idea for companies to try and be reactive to a large amount of complaints in a very short time window? Or should they prioritize the ones that have the most potential impact on their business? This is a nuanced issue.
A key question is, can this platform be used to predict potential complaints before they happen? If businesses could anticipate problems, they might be able to reduce the number of complaints overall. This is a fascinating concept.
Data related to complaints can also be valuable for employees working directly with customers. If they can see patterns or common issues, it can help them be more prepared to handle situations in a way that helps build a better customer experience. There's research suggesting that when companies share data about customer complaints with their employees, it often leads to improvements in customer satisfaction.
An important part of complaint resolution is the follow-up. It's a chance to check in with the customer and make sure they're satisfied with how the issue was resolved. Taking the time to follow up with customers shows that you're invested in maintaining a long-term relationship.
Martinez's work isn't limited to a single industry. It appears that her platform is meant to be adaptable, and there are many industries where businesses could benefit from a more standardized approach to handling customer issues. This is particularly relevant in areas where customer experience is paramount. It's still early days, but the potential benefits are certainly there.
Predict, Trade, and Profit: Decentralized Marketplace for Startup Outcomes and Alternative Assets (Get started for free)
More Posts from predily.io: