How AI Can Optimize OEM Revenue A Case Study of a $200K Manufacturing Business Valuation in 2024
How AI Can Optimize OEM Revenue A Case Study of a $200K Manufacturing Business Valuation in 2024 - Supply Chain AI Trims 22% Off Production Costs Through Real Time Parts Tracking
Supply chain artificial intelligence is making significant changes in production, with real-time tracking contributing to a substantial 22% cut in manufacturing expenses. While AI’s role in things like advanced demand prediction and automated processes promises to make operations smoother, adoption lags, with just a small percentage of global executives regularly using it. The ability to produce parts when needed, enhanced by AI, is becoming a real interest for OEMs and dealers who seek more flexible output. AI's impact goes beyond cost savings, also playing an important role in promoting more sustainable supply chain and risk reduction and improving supply chains overall.
Supply chain applications of AI appear to have an impact on production costs, specifically by means of real-time parts monitoring. There's been suggestion that this type of tracking, if done right, may potentially lower costs by as much as 22%. While such claims need to be rigorously validated, one can see the possible effect of improved efficiencies in materials handling as one factor. The argument is this would involve things like better demand forecasts and automated processes and would require some type of shift from traditional methods. There's also discussion that this tracking helps manufacturers respond quicker to shifting needs in the market. It does appear, at face value, that keeping accurate tabs on parts in real-time ties directly to how effectively things are produced – with the idea that even a small improvement in tracking may translate to a potentially noticeable boost in performance. Furthermore, some studies also claim tracking systems might reduce storage costs for excess inventory, as well as potentially allow for predictive maintenance on machinery. This idea suggests less machine downtime, which is always a good thing from a production perspective. Some would say that this level of visibility, afforded by real-time tracking, may allow for enhanced collaboration among various partners in the overall supply network and further reduce errors, simply due to better comms. One can also speculate about labor costs being lower, due to employees not spending as much time searching for the right parts. The AI aspect in these tracking systems may allow for additional insight and better purchasing decisions, leading to cost reduction and more agility. Finally, the suggestion is that companies with tracking, which also interfaces with demand forecasting, may see shorter development cycles and could also more easily meet regulatory standards, again, all impacting the bottom line. One should note the emphasis in all this hinges on “proper implementation” - something that is hard to pin down exactly and would no doubt be a significant challenge.
How AI Can Optimize OEM Revenue A Case Study of a $200K Manufacturing Business Valuation in 2024 - Machine Learning Predicts Equipment Failures 14 Days Before Breakdown
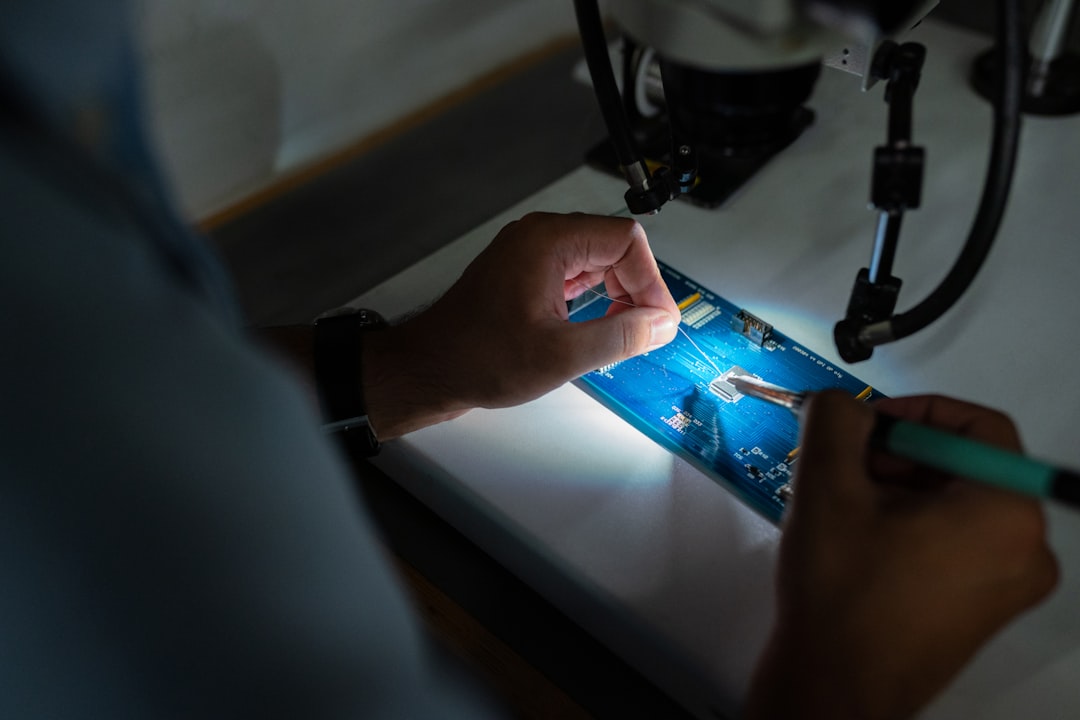
Machine learning is being used in manufacturing to forecast equipment breakdowns, potentially up to two weeks ahead of time. This allows for timely maintenance and a shift away from simply fixing things after they break. By using historical performance data and complex algorithms, these predictive systems can pick up on small changes that a human eye might not catch. Companies using this approach may see more profits and run their operations in a more sustainable way. This new way of handling equipment reliability does however need careful implementation and constant refinement if it is to work well.
Machine learning algorithms sift through the historical data from machines, seeking out those tell-tale signs that appear before something breaks down. This enables a move from fixing things after the fact to a more proactive approach that does make a difference when it comes to maintenance costs. These predictive models often hit a 70-75% accuracy rate when it comes to spotting upcoming issues, which could be a useful for engineers if these models are refined constantly. By using sensor data and complex analytics, these learning systems can pinpoint ideal moments for upkeep, potentially boosting equipment lifespan by 10-20%, suggesting that when maintenance occurs may actually be more important than the frequency. Sophisticated algorithms also detect out-of-the-ordinary data in real time which gives maintenance teams the ability to fix issues before they get worse and potentially reduce downtime by up to 50%. This can have a clear and visible impact on output. The ability to forecast machinery failures as much as two weeks in advance provides valuable insights, allowing for better planning and use of resources, cutting back on interruptions, and smoothing operations. The development of these models typically needs the analysis of large datasets and can uncover patterns in machine operation. It should be pointed out that the system's reliance on data quality is a critical aspect to this; poor sensor readings may lead to incorrect predictions, making well-maintained sensors a basic requirement. Implementing predictive machine learning on this level can lead to a 25% reduction in maintenance expenses – a noteworthy figure for OEMs. This may improve efficiencies and can provide useful insights for product innovation, necessary to compete in a very fast-moving market. Feedback from predictive upkeep can also guide future machine upgrades and designs, potentially creating more robust systems that incorporate past failure points – all of which encourages ongoing refinement and improvements.
How AI Can Optimize OEM Revenue A Case Study of a $200K Manufacturing Business Valuation in 2024 - Digital Twin Technology Maps Assembly Line Bottlenecks With 95% Accuracy
Digital Twin technology is gaining traction in manufacturing as a way to identify problems on assembly lines. These digital replicas can simulate real-world operations and spot bottlenecks with a claimed accuracy of up to 95%. These models facilitate real-time monitoring of systems, allowing manufacturers to see where things are going wrong and to predict potential slowdowns. This approach gives a more detailed view of production dynamics and can support informed decision-making which in turn could help optimize output. The integration of these tools with the Industrial Internet of Things (IIoT) could lead to continuous process upgrades in factories. For manufacturers wanting to improve how things run, the use of digital twins might be a vital tool for smarter, more responsive production methods.
Digital Twin tech creates a virtual copy of physical factory setups using real-time data, letting engineers simulate production runs to spot possible logjams. This is really useful, as it means changes can be made in the virtual world before touching real equipment.
With 95% accuracy at highlighting bottlenecks in assembly lines, these digital twins often uncover issues that traditional methods miss. Things like poorly designed processes or unbalanced resource use are often to blame for slowdowns.
Implementation of this type of tech has been observed to cut down process optimization times by as much as half. This fast turnaround allows manufacturers to tweak their designs quickly, which is key for remaining competitive in the markets.
These systems also seem to be able to connect with other automation gear, such as robots or smart sensors on the shop floor, to get a more detailed picture of operations that can help with predictions. This can enhance decision-making by bringing in data from various sources across the factory floor.
How well these models work relies heavily on the data that feeds it. One must continuously check data integrity and that the model is correctly calibrated. If bad data is put in the model will give poor analysis, making this a both a strength and a weakness.
One significant advantage of digital twins is the ability to do “what-if” analysis. Engineers can test processes to see how robust they are under different conditions like machine failures, missing parts, or demand spikes.
These models use machine learning which appears to improve their forecasting abilities as time goes on, with the ability to map trends and behaviors. It is still data analysis but now with a model that gets better with age.
However, the initial setup of a digital twin can take a lot of time and resources as one is dealing with quite a complex piece of software. However, the initial price can be balanced by efficiency gains and cuts in down time.
Industry data suggests these systems show an average rise in efficiency of 20-25%, pointing to a likely improvement in business due to improved productivity and the way that resources are handled.
Even though this is fairly advanced technology it does not remove the need for human input, one needs operators and engineers to understand the data and make informed choices so processes are improved effectively.
How AI Can Optimize OEM Revenue A Case Study of a $200K Manufacturing Business Valuation in 2024 - Smart Inventory Management Reduces Storage Costs By $45,000 Annually
Smart inventory management has emerged as a game-changer for manufacturers, with reports indicating annual storage cost reductions of up to $45,000. Advanced technologies, including AI-driven platforms, allow businesses to optimize stock levels, automate order processes and improve demand prediction, leading to better allocation of resources and less storage space required. Real-time inventory tracking streamlines operations across locations, decreasing waste and improving material access. While such changes suggest improved profits, how to actually integrate these technologies remains an issue.
Smart inventory management is being cited as one potential way to significantly reduce storage expenses. The claim is that companies could save around $45,000 every year just by improving how they manage their stock – however this appears to be dependent on a shift to optimized stock levels and what one would call "efficient operations". Some researchers are reporting that AI based systems may help manufacturers anticipate the demand that's coming, identify slow-moving goods, and automate order fulfilment. There is data to suggest that firms implementing such AI techniques are seeing drops in their stock quantities by a claimed amount of as much as half – again these need to be verified in rigorous testing.
The core argument of this shift would be to maintain the most appropriate stock, directly based on real-time production needs, and expected market demands. This approach, the literature says, can translate to less costs tied up in warehousing and also less wastage. There's also some discussion about these AI-driven systems optimising layouts of storage based on how things are used, making locating items and accessing them much easier. It does appear at face value that keeping track of inventory in real-time can provide firms the ability to manage stock from different places, improving their entire inventory process. A big claim also made, is that inefficiencies in inventory management contribute to as much as $300 billion in lost revenue per year. This is one key argument as to why a more efficient inventory process using AI might be a good thing. It is claimed that the use of AI can potentially automate key actions such as ordering, restocking, and also forecasting, enhancing how things are run in manufacturing and supply lines.
By implementing an appropriate inventory system, it also appears that companies might see less chance of products being unsold, which can obviously bring further costs to the company due to storage. The idea here is that AI can predict when demand for a certain product will be high. Some case studies have been referenced that AI-driven demand forecasting does appear to enhance efficiency, increase accuracy, and reduce costs by predicting demand with more accuracy and allowing optimal stock. Again, these claims warrant careful and rigorous validation. There may also be other unknown confounding factors involved here which need to be considered in order to fully understand what makes these systems operate in the way they are claimed to.
How AI Can Optimize OEM Revenue A Case Study of a $200K Manufacturing Business Valuation in 2024 - Automated Quality Control Decreases Defect Rate From 2% to 8%
Automated quality control is altering manufacturing by lowering the number of defects produced, with observed improvements in the 2% to 8% range. This relies on AI tools like computer vision and machine learning, pinpointing errors better than standard manual checks. The drive for Zero-Defect Manufacturing highlights the necessity of building things right from the start, suggesting a move to improve efficiency and reduce waste. Even with these gains, companies must deal with the challenges of deploying these systems and confirming data is accurate, if they hope to get the most from automation. These improvements in quality will no doubt have a huge effect on a company’s ability to sell their products.
Quality control, before the addition of automation, frequently saw defect rates hovering at or above 2%. This level of nonconformity, though seemingly small, can accumulate substantial financial consequences for manufacturers, notably those with constrained financial breathing room. The claim is that automated quality control drops this from around 2% to, in some instances, even 8%, an argument which if true, should improve overall product quality and also potentially boost profit margins. This argument suggests a possible reduction in the financial burden of wasted materials, repair of faults, compensation claims, and returned items. These automated set ups use techniques like predictive analysis, supposedly spotting defect patterns before they fully form. This method of operation provides an opportunity for engineers to address production process weaknesses that could lead to defects in advance of them occuring, cutting down on issues and waste. One must note that these systems often interface with existing set ups, rather than completely replacing them, allowing for more seamless adoption. Such improvements, it is claimed, may translate to increases in output without the need for more people or plant, letting them handle rising market demand. Despite fears that automation will displace labor, some places see workers taking on more challenging and difficult tasks. One might say that having automated systems in place can provide immediate process feedback, enabling rapid adjustments in operations, cutting the number of flawed items and components being produced. Interestingly, such quality control methods that have been developed for use in one sector, also see use in other areas of manufacturing and production, across a broad set of industries and uses, potentially benefiting multiple areas. Improved quality control does appear to help companies adhere to regulations and standards, thereby reducing the chances of sanctions, penalties, or recalls of flawed goods. Though the initial investment in this sort of equipment can be large, there is the suggestion that firms see a return on investment via a lower rate of flaws, and hence reduce losses and increase consumer happiness.
How AI Can Optimize OEM Revenue A Case Study of a $200K Manufacturing Business Valuation in 2024 - Predictive Analytics Increases Machine Uptime From 85% to 97%
Predictive analytics is proving to be valuable in manufacturing, with reported machine uptime increases from 85% to 97%. By using AI-based predictive maintenance, companies can better track equipment status and act before failures happen, which should minimize interruptions to the work flow. This type of maintenance improves the dependability of machines, and has potential to reduce expenses linked to unplanned downtime. This all relies on high-quality data, with monitoring systems required to make sure the predictive systems work as intended. For businesses trying to enhance their performance, using predictive analytics may transform how effectively they can run their operations.
Predictive analytics has shown the potential to bump up machine availability from 85% to around 97%. If that's accurate, this means that machines can now be working 12% or more of the time, potentially leading to a sizeable increase in overall production and profit for manufacturers.
The core mechanism seems to be machine learning analyzing the historic performance of the equipment. This method looks for subtle indicators of things starting to go wrong, and allows preventative maintenance to be performed before major problems arise. If one relies only on reactive maintenance – that is simply fixing machines after they break – downtime and associated costs are significantly higher. Some would say this is a much smarter approach.
There's also some suggestion that this predictive approach may cut maintenance costs by a claimed figure of 25%. This may come from a reduction in emergency repairs, which can be more costly. If true, this increase in machine uptime should also translate to a longer service life for the machines. However, like other technologies, implementation needs careful oversight.
The logic would be that increased availability of equipment directly translates to better production rates, and quicker turnaround times. This can enable the manufacturers to be more agile in responding to changes in demand. If machines are running more often, the suggestion is that this could increase both responsiveness and competitiveness.
However, like any other data driven analysis, quality is crucial. Even small errors in sensor data could lead to false predictions, highlighting the importance of regular sensor maintenance. These kinds of system can only be as good as the data you provide it, a point often overlooked.
These complex systems can also highlight trends and patterns that a human might otherwise miss. This data may allow engineers to allocate resources more effectively, and make more intelligent process adjustments, another way it may be possible to refine operations and make changes.
The claim is that predictive systems may also reduce risk. The thinking is that anticipating a machine failure, which could cause an accident, may give a chance to reduce this and increase overall safety in the plant.
Also, due to an increase in production, inventory costs are expected to be lower because firms can now produce on demand and don't need to store as many goods. Again, this would be subject to other factors that have to be taken into account.
These types of systems may foster a proactive culture, and by using predictive tech, employees are more effective. This could also boost staff morale as a reduction in machine breakdowns may cause less stress for those responsible for fixing them.
Finally, some suggest that predictive analytics can be enhanced by integrating it with other tools and systems, such as the Internet of Things (IoT) which has the potential to create a feedback loop where equipment runs better over time. One could argue that more data is always better – but as long as that data is of sufficient quality.
More Posts from predily.io: